Harish Haresamudram
PhD student in the Computational Behavior Analysis Lab, at Georgia Institute of Technology
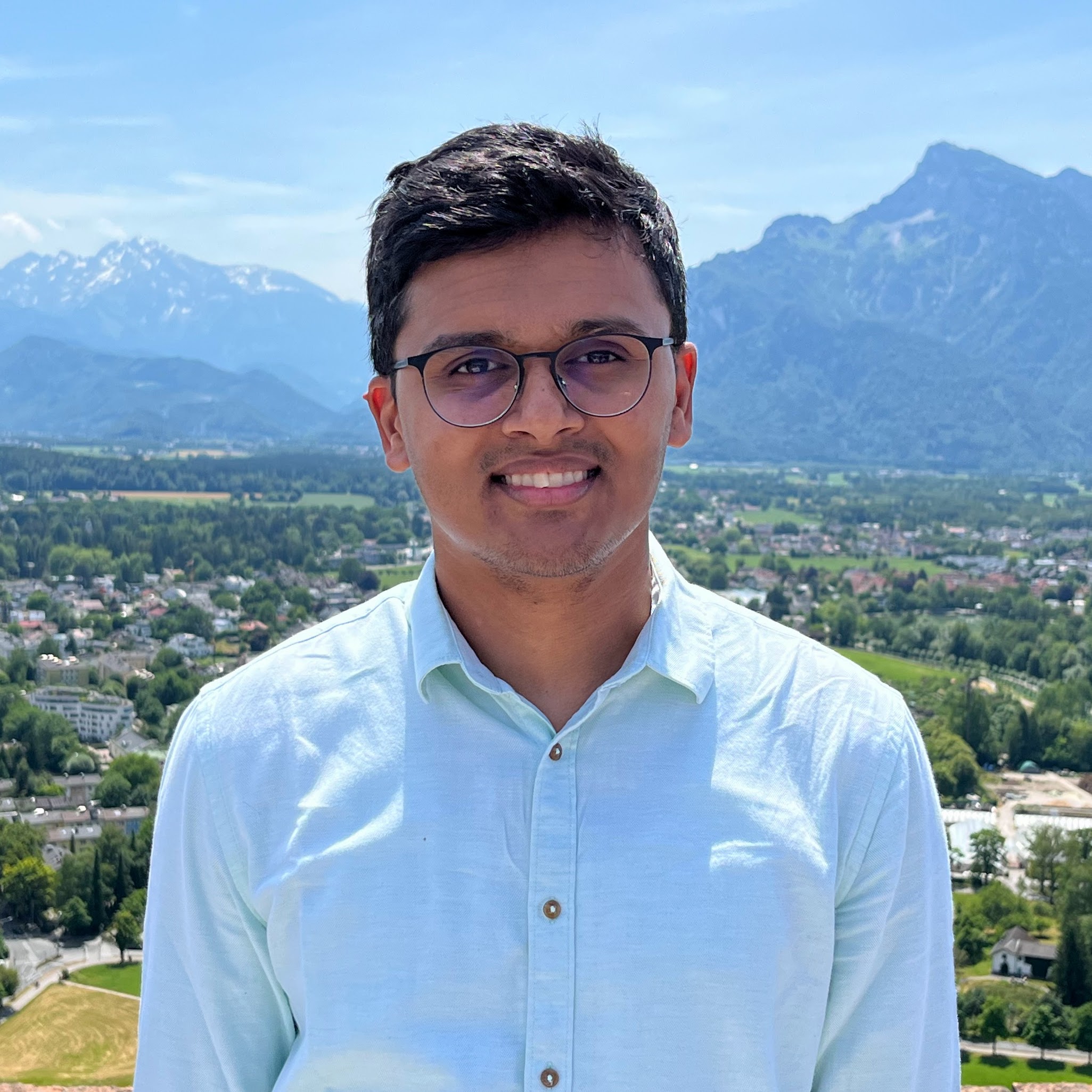
I am a final year PhD student with the School of Electrical and Computer Engineering (ECE) at Georgia Institute of Techology, Atlanta. I am advised by Prof. Thomas Ploetz and Prof. Irfan Essa. I received my Master’s degree in May 2019 from Georgia Tech, where my thesis studied the role of representations in human activity recognition using wearables. I was advised by Prof. Thomas Ploetz and Prof. David Anderson. I have been supported by funding from the National Science Foundation (through the AI-CARING Institute), Optum AI, and Google.
Research
My research broadly involves learning representations for time-series data, with a special focus on developing techniques that require minimal supervision. I develop unsupervised and self-supervised learning algorithms for data from wearable sensors, including accelerometers, gyroscopes and intertial measurement units (IMUs). Subsequently, I use such representations to analyse human behavior, through movements and activities..
News
Dec 04, 2024 | Our paper: Limitations in Employing Natural Language Supervision for Sensor-Based Human Activity Recognition–And Ways to Overcome Them has been accepted to AAAI 2025! Looking forward to presenting it in Philadelphia! |
---|---|
Nov 11, 2024 | Based on our two Ubicomp tutorials on HAR, we release a new paper: Past, Present, and Future of Sensor-based Human Activity Recognition using Wearables: A Surveying Tutorial on a Still Challenging Task on ArXiv! It contains the history of HAR from heuristic-based features to the current trend of multimodal training, accompanied by a code walkthrough of standard self-supervised methods! |
Oct 06, 2024 | Organized the second tutorial on Solving the Sensor-based Activity Recognition Problem (SOAR) in Ubicomp 2024! |
Aug 21, 2024 | New paper is out on ArXiv! We show that zero shot prediction of activities through CLIP-like training on a large background dataset results in poor HAR! See: Limitations in Employing Natural Language Supervision for Sensor-Based Human Activity Recognition–And Ways to Overcome Them |
Jan 01, 2024 | We show that learning discrete representations of human movements leads to better sensor-based Human Activity Recognition (HAR) in Towards Learning Discrete Representations via Self-Supervision for Wearables-Based Human Activity Recognition. |
Selected publications
- Towards Learning Discrete Representations via Self-Supervision for Wearables-Based Human Activity RecognitionSensors, 2024
- Investigating enhancements to contrastive predictive coding for human activity recognitionIn 2023 IEEE International Conference on Pervasive Computing and Communications (PerCom), 2023
- How Much Unlabeled Data is Really Needed for Effective Self-Supervised Human Activity Recognition?In Proceedings of the 2023 ACM International Symposium on Wearable Computers, 2023
- Assessing the State of Self-Supervised Human Activity Recognition using WearablesProceedings of the ACM on Interactive, Mobile, Wearable and Ubiquitous Technologies 6.3 (2022), 2022